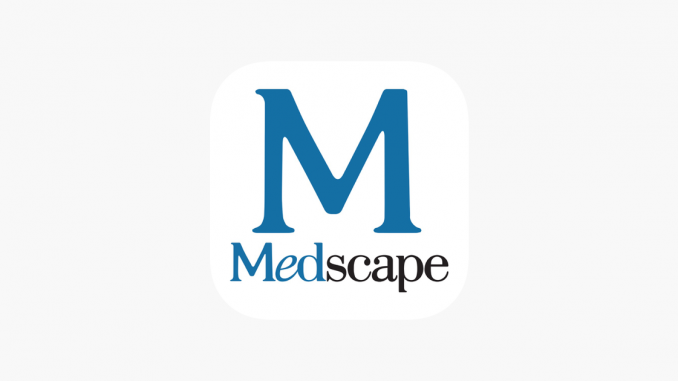
Ricki Lewis, PhD August 05, 2019
Machine learning algorithms predicted, with 90% accuracy, the training level of 50 individuals on the basis of their skills performing virtual neurosurgery, according to findings published August 2 in JAMA Network Open.
As a subset of artificial intelligence, machine learning (ML) uses data generated from “training” situations and scenarios to guide predictions and decision making in other circumstances. It is affecting an ever-increasing variety of fields, from predicting financial meltdowns, to guiding streaming services in developing new programming, to revealing hidden patterns in fine art. ML lies behind Siri’s recognition of the speech patterns of an iPhone user and peppers Facebook feeds with those annoyingly spot-on ads.
In the medical arena, ML is refining diagnostics, with entire issues of medical journals devoted to the approach. In the new study, Alexander Winkler-Schwartz, MD, of the Neurosurgical Simulation and Artificial Intelligence Learning Centre at McGill University in Montreal, Canada, and colleagues applied the eclectic strategy, dubbed “surgical data science,” to match the technical skills of 50 volunteers to the standard levels of expertise associated with the points on the pathway toward becoming a neurosurgeon.
The potential value of ML in evaluating surgical skill is twofold: revealing unrecognized patterns in performance that can perhaps be applied to minimize error, and grouping participants according to technical ability, which can inform training practices and evaluation.
The prospective, observational case series investigation identified specific metrics used in a virtual reality surgical procedure that enable an algorithm to accurately classify participants by level of expertise: expert (neurosurgeon), senior (neurosurgical fellows and senior residents), junior (neurosurgical junior residents), and medical students.
At McGill University from March 2015 to May 2016, nine women and 41 men (mean age 33.6, standard deviation, 9.5 years) resected five virtual primary cortical brain tumors through microscopes, removing tissue with an ultrasonic aspirator. The two-handed procedure required peeling back the pia and cauterizing blood vessels. It simulated removal of cancerous brain tumors as well as those that cause epilepsy, with the goal of preserving surrounding structures.
Participants were given 3 minutes to complete the procedure and did so five times. The group consisted of 14 neurosurgeons, four fellows, 10 senior residents, 10 junior residents, and 12 medical students.
The 270 metrics the study assessed fell into four principal domains: movement associated with a single instrument, with both instruments, the force the instruments apply, and tissue removed or bleeding. The assessments included specifics such as jerking movements, change in bleeding speed, converging and diverging of instrument tips, and change in the volume of the tumor.
The four algorithms deployed had accuracies of 90%, 84%, 78%, and 76%. Overall the strategy misclassified two neurosurgeons, one senior resident, and one junior resident, and three of the four algorithms misclassified a single medical student as a neurosurgeon.
The investigators conclude, “We found that the best-performing machine learning algorithm used as few as 6 performance metrics to successfully classify 45 of 50 participants into 1 of 4 groups of expertise…These findings suggest that algorithms may be capable of classifying surgical expertise with greater granularity and precision than has been previously demonstrated in surgery.”
The approach can be used in other surgical procedures, the researchers say. George Shorten, MD, PhD, from the Department of Anaesthesia and Intensive Care Medicine at Cork University Hospital in Ireland, agrees in an accompanying commentary. “The authors’ work prompts wider consideration of how to apply artificial intelligence to human behavior in medicine, particularly to the performance of technical tasks…The authors insightfully point out the potential value of explainable artificial intelligence in the setting of training humans on technical skills.”
The beauty of the ML approach, Shorten adds, is that associations strengthen as data accrue, building powerful predictive tools. But a limitation is that even 270 metrics “still represent a small subset of all possible motion or position metrics that might represent expert or novice performance.”
Shorten then points out that surgeons may arrive at the same outcomes via different routes, and that the ML approach may not capture such individual combinations of strategies and skills. Adding assessments of psychomotor and visuospatial abilities and handedness, he adds, may help to account for individual steps in the choreographies of surgery.
Winkler-Schwartz and coauthors Recai Yilmaz, Rolando Del Maestro, Nykan Mirchi, and Nicole Ledwos have a patent pending for a surgery training platform. Shorten has disclosed no relevant financial relationships. JAMA Network Open. Published online August 2, 2019.